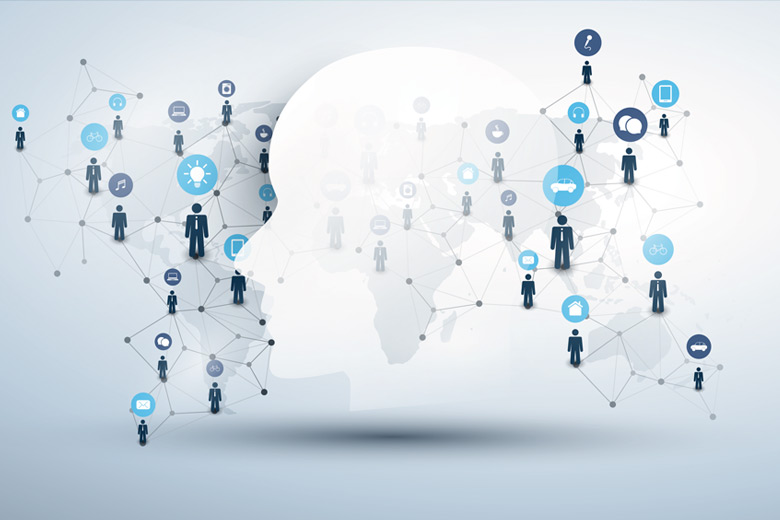
Dive in and find out how the evolution of AI tasks, neural networks, advanced research, and applications are paving the path to a stronger artificial intelligence (AI) – even as some challenges envelope this road.
“It’s not about the shoes. It’s what you do in them”.
[ez-toc]This punch-line of a trendy sneaker brand- could not have been more relevant than today. Contrary to what many sneaker-heads would opine, the actual value of a good shoe lies in its on-ground sturdiness. It’s all about being rugged in all weathers – whether AI or Leather.
Of all the breakthroughs, AI is not supposed to gather dust in a closet. And its unlimited potential hinges a lot on the user of artificial intelligence (AI) – how and where that user applies it. So has AI been advancing well on the street? Has it gotten off the kerb and explored real-life applications and research areas? And if so, what kind of tasks and adoption curves have we seen so far? Let’s test these shoes.
- Real life applications of research areas
- Task classification of artificial intelligence (AI)
- Artificial intelligence (AI) and neural networks
- Conclusion
1. Good shoes take you to good places! Real-life applications
Artificial intelligence (AI) is expanding in many dimensions of powerful research and applications – especially in the last few years. Enterprises- across almost every vertical type- are experimenting with artificial intelligence (AI) to accelerate decisions, improve precision, achieve customer-centricity, and gain new efficiencies.
Deloitte’s State of AI report 2020 outlines how AI benefits are multi-faceted and hard to ignore. In a survey of 1900 early adopters across seven countries here, it was seen that:
- 43 percent find it useful to enhance products and services
- 41 percent for optimizing internal business operations
- 34 percent for making better business decisions
- 31 percent for optimizing external business processes
- 28 percent find it helpful to create new products and services
- 27 percent use it to pursue new markets
- 26 percent capture and apply insufficient knowledge
AI’s Acceleration
- Expert levels of tasks
- Hi-end research work
- Applications in many verticals
- Wider and faster adoption
- New investments and bold traction
As for research, it is hard to deny the increasing attraction which AI manifests today. In 2019, 65 percent of graduating North American PhDs in AI went into the industry—this was considerably up from 44.4 percent in 2010. It reiterates the more significant role the industry has begun to play in AI development (AI Index Report 2021 by Stanford University and HAI). We also saw that the number of AI journal publications went up by 34.5 percent from 2019 to 2020—this was relatively better than what we saw from 2018 to 2019 (19.6 percent). In just the last six years, the number of AI-related publications on arXiv has blossomed over six-fold, from 5,478 in 2015 to 34,736 in 2020. It is also causing researchers to invest in technologies for the detection of generative models. The DeepFake Detection Challenge data, for instance, indicates how well computers can distinguish between different outputs.
Plus, An AI Index survey conducted in 2020 suggests that the world’s top universities have pumped their investment in AI education over the past four years. In the European Union, the vast majority of specialized AI academic offerings are taught at the master’s level; we see that robotics and automation is the most frequently taught course in the specialized bachelor’s and master’s programs, while machine learning (ML) is emerging as a predominant one in the specialized short courses. If this is the scenario on the research side, the business side translation is quite strong too. The advent of AI is attaining higher degrees of scale, sophistication, and impact, as visible already in industries of enormous complexity, stakes, and difficulty levels.
According to the AI Index Report 2021 by Stanford University and HAI, AI investment in drug design and discovery increased significantly: “Drugs, Cancer, Molecular, Drug Discovery” received the most significant level of private AI investment in 2020, with more than $13.8 billion – this was 4.5 times higher than 2019. Also, AI systems can now compose text, audio, and images to a sufficiently high standard. In fact, humans have a hard time telling the difference between synthetic and non-synthetic outputs for some constrained technology applications. Scientists have started to use ML models to learn representations of chemical molecules for more effective chemical synthesis planning.
2. Don’t change the foot, if the shoe doesn’t fit! Task classification
Let’s just take the tasks that AI does- today. Based on this characteristic, we can slice AI into Mundane, formal, and Expert tasks. The Mundane ones incorporate areas like perception, vision, speech, natural languages, understanding, reasoning, translation, and robot control. The Formal ones comprise complex mathematical, logical, and geometrical areas. Finally, the Expert ones are where we can bring in financial analysis, diagnosis, engineering, simulation, and scientific experiments.
3. Artificial intelligence (AI) and neural networks
An interesting pillar of AI’s explosive rise is the progress made in the area of neural networks. Put simply- these networks run the algorithms that form the core of any AI model. They are loosely constructed on the map of human biological networks in the brain. They work by drawing reference from the collection of units or nodes called neurons – which model the neurons in the brain. They use this to form a system of hardware and software which is shaped on these highly interconnected processing elements (neurons). Further, an artificial neural network (ANN) can evolve from the fundamental – and it can be used to comprehend the relationship between datasets to generate a desired output. To arrive at that, the system simulates a human brain via some deep learning technologies to solve complex pattern recognition or signal processing problems.
Here are some applications of neural networks:
- Weather prediction
- Handwriting recognition
- Fraud detection
- Risk analysis
- Oil-exploration data analysis
- Facial recognition
- Speech-to-text transcription
- Banking- credit and loan application evaluation
- Predictive analytics for loan delinquencies
- Transport- power routing systems, truck brake diagnosis systems, and vehicle scheduling
- Healthcare- cancer cell analysis, advanced diagnostics and design
- Customer support
We can notice that as AI models and applications explode in growth, there is a corresponding rise in the use of neural networks. In the estimates of Allied Market Research, the global neural network market stood at $14.35 billion in 2020 and can climb to $152.61 billion by 2030. Furthermore, as per MarketsandMarkets, the global artificial neural network market can show a spurt from $117 million in 2019 to $296 million by 2024.
The fast state of adoption can be understood better by getting closer to the breakdown of tasks that AI handles. Of course, they differ in their complexity and tech maturity. But as we will reckon ahead, AI is evolving quickly here.
In the AI-Index report from Stanford, what also emerges is a trend of AI gaining traction and confidence in the expert segment of tasks. Computer vision is now industrializing rapidly. Companies are investing increasingly large amounts of computational resources in training computer vision systems- this is happening faster than ever before. Then technologies for use in deployed systems—like object-detection frameworks for analysis of still frames from videos—also show AI deployment and maturity. We can also observe notable progress in natural language processing (NLP). That has yielded AI systems with significantly-improved language capabilities to have a meaningful economic impact on the world.
The McKinsey State of AI 2021 survey shows that AI adoption continues to grow and that the benefits remain significant— though, in the COVID-19 pandemic’s first year, they were felt more strongly on the cost-savings front than on the top line. Moreover, as AI’s use in business becomes more common, the tools and best practices to make the most out of AI have also become more sophisticated. Fifty-six percent of all respondents report AI adoption in at least one function, up from 50 percent in 2020. Notably, AI adoption increased most at companies headquartered in emerging economies, including China, the Middle East, and North Africa: 57 percent of respondents signified strong adoption, up from 45 percent in 2020. And across regions, the adoption rate is quite huge at Indian companies, followed closely by those in Asia–Pacific.
AI adoption is becoming common in areas like service operations, product and service development, and marketing and sales. Popular use-cases are service-operations optimization, AI-based enhancement of products, and contact-center automation – do note that the most significant percentage-point jump has been in the use of AI in marketing-budget allocation and spending effectiveness.
AI’s Hiccups
- Lack of quality AI talent
- Inadequate upskilling and reskilling
- High demand for new AI roles
- Need for AI builders as well as translators
Leveraging the ultimate potential of AI needs a lot of work in many areas. If we look at some obstacles enterprises face before/with AI adoption, we reckon that some struggles need particular focus and interventions.
There are a lot of challenges that envelope AI adoption. For example, in Deloitte’s State of AI report 2020, it can be seen that enterprises face several challenges – from integrating AI in the company’s roles and functions (38 percent), to data issues (38 percent), to implementation issues (37 percent), measuring and providing business value (33 percent).
But to top it all, a lot of shoe-horning remains to be done with AI on the aspect of talent. Unless AI is supported with the right skill sets, unless professionals keep reskilling and up-skilling in AI, it would be a futile exercise to have hope in AI. The power and possibilities of AI are pointless if adequate human energy, proficiency, support, and dexterity are not around to steer it.
As per a Global AI Talent Report 2020, the growth rate of specifically AI devs (-68.8 percent) is inverse to that of data scientists (102.7 percent). On the other hand, median growth rates for data analyst, data scientist, and ML engineer roles attained specific stability in 2019, between 1.24 percent and 3.28 percent. ML researcher roles, meanwhile, have a much higher median growth rate of 6.28 percent. Add to this that researchers make up 1.77 percent of the demand measured here, while they only comparatively make up 1.02 percent of the talent supply available to the industry. We can expect that competition will get even tighter for research talent. As a result, more researchers may be drawn out of academia.
The report argues that AI devs need to understand the underlying engineering to fit novel AI tools into the software. The challenging problems to solve in AI software development are why AI has been largely inaccessible to smaller teams who can’t afford both ML engineers and specialized AI devs. The aim to democratize AI with standard, out-of-the-box tools could allow the average developer with some online AI training to implement AI in their software. This is good for smaller teams as it will enable them to put more of their budget towards implementation rather than engineering and still be able to tweak out-of-the-box methods with some customization.
The report also highlights that as AI matures, it will become more pervasive. We will see new specialized roles emerge for managing the new dynamics of AI, but eventually, everyone will need to update their digital skills to collaborate with this new technology. Already, we have seen that most people can grasp the concept of an AI-powered recommendation algorithm and adjust their behavior to affect the algorithm’s output. However, people have the minimal choice and only blunt tools for manipulating an algorithm to their needs. When the different tooling and skill sets standardize along the value chain, it will vastly increase the choice and access to AI technology and engender far more innovation than we have seen with AI software.
To get there, we challenge bridging the gap between proof-of-concept in the lab and real-world deployment. Researchers and engineers play an essential role in helping close that gap, but they cannot do it alone. The institutions that train them have to sharpen their focus on standardizing their tools and processes so that others can more easily strike collaborations with others down the value chain.
The proportionate mix of specialized engineering, technical implementation, and research roles in demand is closely matched with the combination of supply: about 61 percent for implementation roles building the software around AI capabilities, 38 percent for AI engineering roles building the core AI capabilities, one percent for researchers. We do not know the aggregate amounts, but the monthly flows grew steadily in 2019, around 2-6 percent for different job titles. Unsurprisingly, we saw 20-30 percent drops in demand for the relevant job titles during 2020, but both 2019 and 2020 show significant outliers for those entering the scene and persisting through the pandemic. India’s capacity to attract and retain talent in 2018-19 showed -0.183 that were invited and -0.315 that stayed.
A survey by The European Commission recently found businesses identified access to the right skill sets as being the number one impediment to adopting AI. Also -as per a Nasscom report, the demand for highly-qualified, experienced, and technically adept talent in the field of AI and BDA outstrips the current supply. Therefore, there is a severe need to narrow the demand-supply gap by upskilling talent across the AI and BDA occupations. While total job openings stood at about 140K in 2018, they touched about 230K in 2021. But university talent was about 78K in 2018 and projected to hit only some 90K in 2021 – showing a talent-supply gap of approx—140K in 2021. India had an employed talent pool of about 370,000 skilled in AI and BDA in 2018, and if we look at the projected total demand for AI and BDA for 2021, it was around 800,000.
In the Deloitte’s State of AI report 2020, we can see that 68 percent of executives report a moderate-to-extreme skill gap, and 27 percent find the skill gap an extreme one. The top four most needed AI roles are AI builders – this segment entails the need for AI researchers (30 percent), software developers (28 percent), data scientists (25 percent), and project managers (23 percent). Companies also need AI translators to bridge the divide between business and technical staff. This is required at both the front-end and back-end of building AI solutions.
Here the most sought-after professionals are:
- Business leaders (22 percent).
- Change management experts (22 percent).
- User experience designers (21 percent).
- Subject matter experts (20 percent).
4. Conclusion
AI’s potential cannot be realized without adequate support from the talented side. With AI, it looks like the world is at your feet, so it’s all the more vital that you should wear the right shoes. AI is a big turning point for this world – from compelling research areas and unprecedented applications to staggering levels of human augmentation. Let’s make the most of this turn.
As they say- Cinderella is proof that a new pair of shoes can change your life.